Abstract
Artificial intelligence (AI) and machine learning (ML) have promising prospects in the healthcare sector where it is projected to take up some of health workers’ responsibilities and optimize work processes. As of now, AI and ML have found their use in anomaly detection, predictive modeling, and scoring systems. Some of the algorithms that are only emerging in the healthcare sector are already widely used in finance.
The question arises as to how compatible these algorithms with the current needs of the healthcare system and what possible problems may occur when validating them. Scoring systems in medicine give rise to a reasonable doubt concerning their ethicism and precision. The validation of predictive modeling and anomaly detection largely used in finance may be challenged in the light of new scientific findings that require ongoing readjustment. Lastly, the healthcare sector suffers from the lack of cohesive shared databases, which would slow down validation and implementation of the new algorithms.
Intorduction
One of the greatest challenges of modern healthcare is the need to handle and process big data in order to receive interpretable and applicable results. The new information to be managed includes consumer, patient, physical, and clinical data whose volume has grown so vast that it can no longer be analyzed manually (Raghupathi & Raghupathi, 2014). One way to tackle these new challenges is to introduce artificial intelligence (AI ) algorithms.
AI, otherwise known as machine intelligence, is software that estimates human cognition in the analysis of complex data. Machine learning algorithms are able to recognise patterns in a dataset and create their own logic. As of now, AI in health care has promising perspectives, but its application is likely to be gradual. It may also not come as a surprise if the implementation will be met with initial resistance, in which case it is imperative to clarify the rationale for using AI in medicine.
First, artificial intelligence algorithms for health data are needed in the workplace. Healthcare professionals are typically only trained mathematics and statistics at the surface level, and being confronted with big data in the workplace often leads to frustration. Besides, health care is becoming overwhelmingly evidence-based, which implies that relying solely on one’s formal training and professional opinion suffices not (Buch, Ahmed & Maruthappu, 2018). Health workers often cannot afford analyzing each case individually let alone make foregoing conclusions regarding each outcome. Rapid scientific advances, the increasing population and the growing burden of disease are all pushing the healthcare sector to seek new solutions.
Another rationale behind locating the appropriate methods for handling big data is the growing healthcare expenditures. As Kayyali, Kuiken and Knott (2013) stated in a report for McKinsey & Company, health care has been steadily represented an ever growing share of the US GDP. Back in 2013 when the report was published, the tendency was already seen as fairly alarming. The expenses surpassed the benchmark of $600 billion, amounting to 17.6% of GDP. Five years later, the trend had only picked up the pace. According to the analysis conducted by Sisko et al. (2019), in 2018, Americans spent more than $3.65 trillion on health care. To give this piece of statistics a point of reference, that amount was comparable to the entire GDPs of countries such as Brazil, the United Kingdom, Mexico, Spain and Canada.
The question arises as to what the exponential growth of health information volume has to do with the increased healthcare expenditures. Now, more experts than ever concur that some of the costs can be leveraged or avoided altogether through the application and use of predictive modeling. What this means for the healthcare sector is that the economic paradigm might need to be reinvented. Namely, insurance companies need to prioritise patient outcomes over fee-for-service plans (Dinov, 2016). The new strategy would focus on the long-term perspectives and discard unnecessary treatments. However, predicting the necessity of a particular treatment, patient outcomes and total costs is only possible through processing big data.
Some countries have already tested AI in their healthcare sectors and yielded positive results, even though so far they have been confined to the local level. A prime example would be China that has been leading a revolution in the digital sphere for the last decade. Walker (2018) reports that Health Connect, a Chinese company under a large medical insurance provider, has successfully made a case for AI. One fourth-tier town in China was the first one to experience the impact of predictive model and big data analytics to outpatient chronic disease management.
Before the intervention by Health Connect that took over took over the management responsibility, the local Social Health Insurance (SHI) office used to see 20% annual increases in health expenditures. In nine months after the new technology was introduced, the trend had plummeted to a negative 1.2%.
Now that the rationale for the use of AI in health care has been clarified, it is essential to outline the scope of its application as well as the medical subfield that it can aid the most. In cardiology, the promise of artificial intelligence and machine learning is to provide a cardiologist with a set of tools that would lighten their workload and make their practice more robust (Johnson, 2018). The field of cardiology would benefit immensely from AI-implementation for several reasons.
First, the burden of heart disease in the United States is growing at an alarming rate. According to the data provided by the Centers for Disease Control and Prevention (2017), every year, heart disease kills around 610,000 people. Heart disease accounts for every fourth death in the country and in the last few decades, has become the leading cause of mortality in both genders (Kadi, Idri, & Fernandez-Aleman 2017). Annually, about 735,000 Americans suffer from a heart attack; for two-thirds of them, it is the second heart attack, and for one-third, it is the third (Mozaffarian et al., 2015).
Artificial intelligence offers a proactive approach that would prioritize prevention as opposed to handling the consequences of disease. As seen from Graph 1, the fastest growing area of application for AI is diagnostic imaging, which also largely pertains to cardiology (Jiang et al., 2017). The cardiological field primarily employs diagnostic imaging in the form of such non-invasive procedures as ultrasound, magnetic resonance imaging (MRI), computed tomography (CT), or nuclear medicine imaging with PET or SPECT (Kwong, Jerosch-Herold, & Heydari, 2018). Another area that is related to cardiology – monitoring – has yet to gain enough traction, as seen from Graph 1.
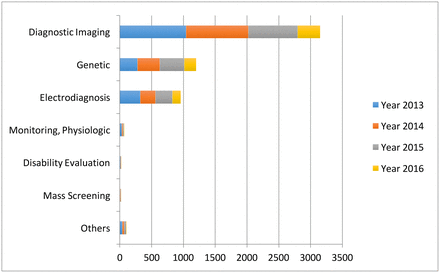
As of now, heart disease is researched well enough to define the main causes, some of which may as well be modifiable. One cause is old age – a criterion that can help to form risk groups within patient databases (Stubbs, Kotfila, Xu, & Uzuner, 2015). Other criteria such as lifestyle choices that may lead to the development of heart disease may also be considered for group formation as well as medical recommendations (Fatima, Anjum, Basharat & Khan, 2014). Apart from making predictions, the use of AI may mean faster and more personalised care – something that is now increasingly demanded by patients (Johnson, 2018). In summation, artificial intelligence and machine learning may as well increase the overall efficiency of the healthcare sector while customizing individual treatment plans.
Even though artificial intelligence and machine learning form a relatively young subfield of study within mathematics, statistics and computer science, there have already been developed various solutions. The question arises as to what solutions would be the most appropriate for health care and whether it is possible to “borrow” some algorithms from other fields and industries. The present paper theorises the possibility of integrating some of AI algorithms recognised in financial technology (so-called fintech) into health care.
The fintech sector has been gaining plenty of traction in the last few years. As compared to the healthcare sector, one may assume that financial companies, especially new entrants, have more flexibility. Moreover, the number of financial companies cannot be limited as opposed to healthcare providers – a market with an arguable higher entrance barrier. According to Columbus (2019), next year, as many as 77% of incumbent financial institutions will focus more on internal innovations such as introducing artificial intelligence and machine learning.
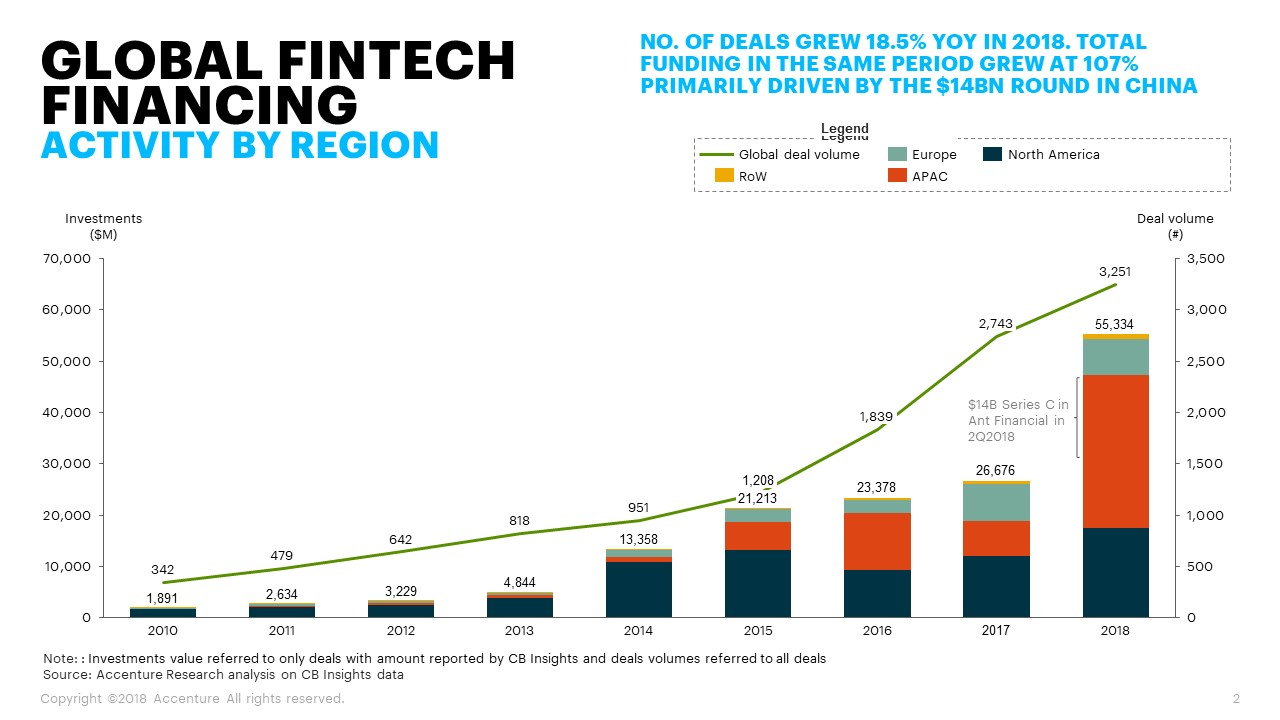
The business environment predisposes the fintech to experiment and capitalise on innovation. According to Andjelic (2019), in 2018, global fintech companies acquired around $111.8 billion in investments. Graph 2 showcases an exponential growth of investment across the board. Apart from that, the industry is characterised by high competitiveness, which pushes its players to get ahead of their contenders by applying new solutions. All points taken into consideration, the key hypothesis for this paper is that the majority of fintech-related AI algorithms can find their place in the healthcare sector, though through customization and readjustment.
Methodology
The present paper is a literature review that includes the current knowledge about artificial intelligence and machine learning algorithms in finances and healthcare. The review encompasses theoretical and methodological contributions to the subject matter as well as covers substantive findings. All sources used in the present review are secondary sources and do not report any experimental work or new findings. The literature review was conducted in several steps that are presented in Figure 1 below.
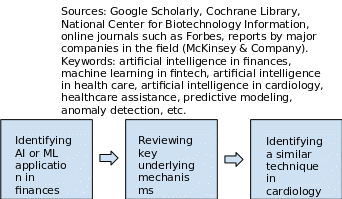
Literature Review
The accompanying survey gives abridges the key points on the utilization of machine learning and artificial intelligence in the financial and healthcare sectors. Each sector shares reasoning for the application of AI and ML algorithms, which is later elaborated to confirm whether the two fields show similar needs. Afterwards, the review covers the most common AI and ML applications in finances and discusses matching practices or opportunities for use in healthcare.
Credit Scoring to Medical Scoring
One of the key applications of AI and ML for big data in finance is developing algorithms for credit scoring. Credit scoring is defined as statistical analysis of data by lenders and financial institutions to determine a person’s creditworthiness (Bahnsen, Aouada, & Ottersten, 2014). For many people in North America and some other regions, access to credit is the key to financial prosperity and upward mobility. Enhancing one’s credit score and keeping it intact are some of the requirements to make such serious life decisions as purchasing a home or a car or starting a new business. In some particular cases, a solid credit score is what ensures employment, decent rental housing and insurance.
A prime example of a system that evaluates trustworthiness of individuals is the United States’ FICO that was first introduced in 1989. As of now, FICO uses a comprehensive scale that spans from zero to 850 (see Figure 2) with good scores starting at around 720. The creators of the FICO system keep the exact formula used for calculating scores a system. The only piece of information available to the general public is the key factors that the formula considers as parameters and their priority when calculating (see Figure 3). Other than that, citizens do not have control over their personal data; they only know what financial behaviour is considered good and will be rewarded by financial institutions.
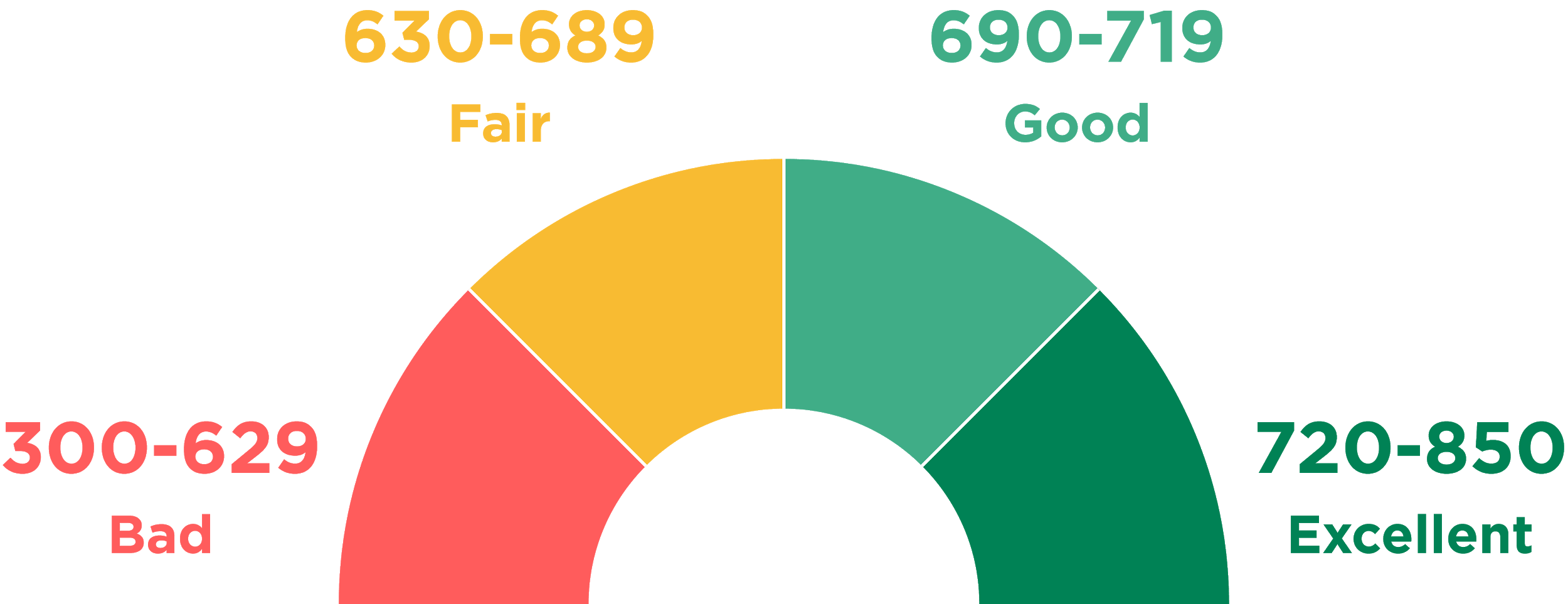
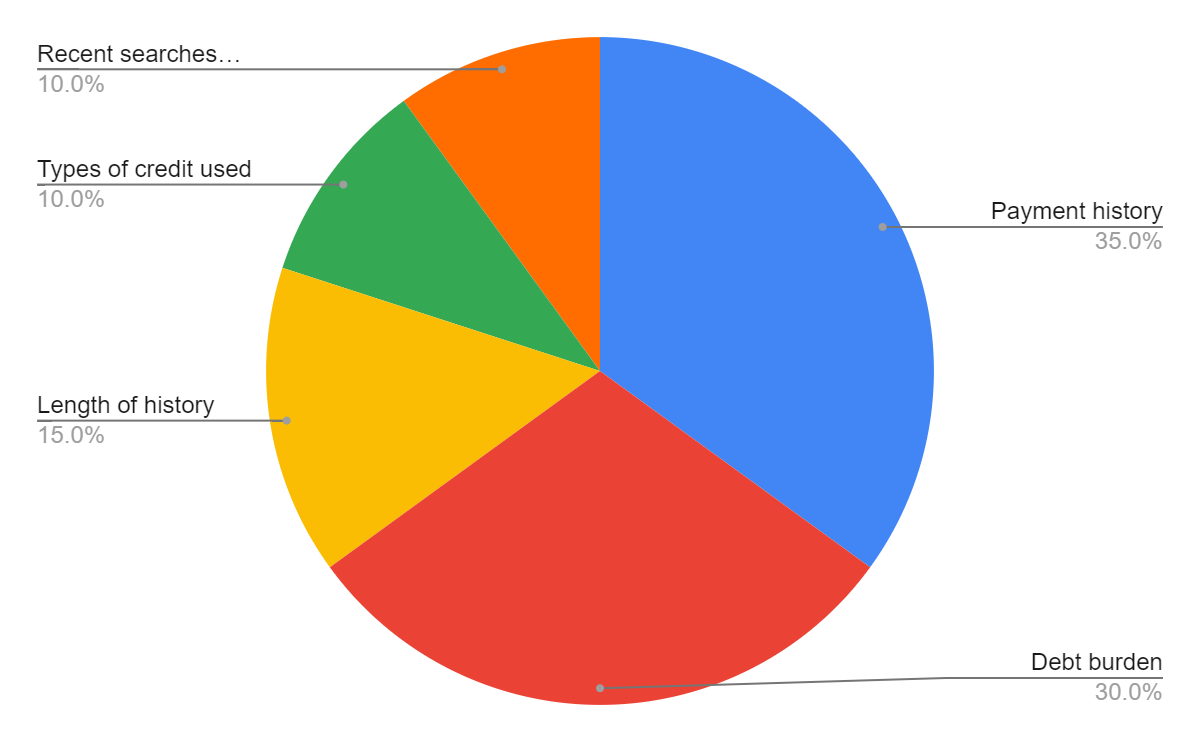
While the public might never know the actual formulas for credit scoring, it is possible to speculate about methods used. From the description of credit scoring systems, it becomes clear that whatever algorithm is applied, it uses multiple, confounding variables. It may only contain those presented in Graph 3 (payment history, debt burden, length of history and others) as well as some other minor properties. In any case, the output of the algorithm is singular, which the exact value describing a person’s credit score.
The best known statistical method for handling many variables pointing to a single outcome is multiple regression (see Image 2 for the generalised formula). Basically, multiple regression is an extension of simple linear regression. The latter is commonly used to define a relationship between two variables with one being independent and the other dependent. In the case of credit scoring, the independent variables are a person’s credit history (broken down into basic elements in Graph 3), and the dependent is the credit score.

In cardiology, the scoring algorithm can be applied to set the health assistance priority system. Simply put, if a patient neglects their health to the point where they constantly face readmissions and need extended treatment plans, their priority in the system is lowered. It may go as far as cutting off the access to cardiology-related services that are otherwise covered by insurance. The logic behind the “medical credit” score would aim at making patients more conscious of their health decisions, which is viable given the major financial incentive – the access to medical insurance. Another way to apply the logic behind credit scoring is to identify risk groups within populations served.
Those who would be assigned to high- and very high-risk groups would be referred for future examination and possible counseling to develop an appropriate disease management strategy. Surely, assessment that concerns matters as serious as human health needs a sophisticated solutions. However, at the point of it existing only as a hypothesis, it suffices to define some variables for later use in multiple regression.
According to the Centers for Disease Control and Prevention (2018), heart disease risk factors can be broken down into three groups: conditions, behaviours and family history. As one may notice, two of the three groups consist of factors that are hardly controllable. For instance, if a person is born into a family with a history of coronary heart disease, the likelihood of the said person suffering from the same condition is increased. However, taking governance over one’s behaviour is a decision to be made by every person. Thus, the independent variables for the ultimate equation would include unhealthy diet, physical inactivity, smoking and alcohol.
The question arises as to how practices established in finance could help to validate scoring algorithms in cardiology. Two possible applications of scoring systems in healthcare have been described, and each of them is likely to require its own validation mechanism. Concerning a system that sets health assistance priorities based on a patient’s behavior and previous history, the efficiency of a model can only be validated in the long-run. The system will prove working if readmission rates for heart disease and other cardiological conditions will be on decline. At the same time, another validation criterion could be a decrease in behaviors that are considered to be negatively impacting heart health.
Tao, Hoffmeister and Brenner (2014) discuss scoring system validation in relation to colorectal neoplasm risk. However, their findings are not that field-specific to prevent them from being used in cardiology. Tao et al. (2014) emphasize the importance of testing a scoring algorithm on a large and diverse population. The researchers point out that many previous studies overrepresented Caucasian males while ignoring other demographics; others had small samples. According to Tao et al. (2014) a large population allows for not only more accurate modeling but also for identifying additional risk factors. To build a multiple regression model, developers typically start with defining independent variables, or risk factors in the case of cardiology. A series of tests may prove some of the factors inadequate and help locate new, more appropriate ones.
Predictive Modelling
Predictive modeling is the process of using existing data to create, process and validate a model that can be applied for forecasting future outcomes. Modeling is one of the key tools used in predictive analytics, a data mining technique seeking to gauge the possibility of a particular event happening in the future. The rapid digitalization of products and services allowed businesses to access data and use it for their purposes. In finance, big data is utilised by businesses to enhance the dynamics of B2C (business-to-customer) relationship. By analyzing historical events, a business is able to make predictions and plan accordingly. Since the data needed for predictive modeling is often too complex and unstructured to be processed manually, the task is often delegated to machines.
As of now, computer science and interdisciplinary fields with which it overlaps knows a variety of predictive modeling tools and formulas. The most commonly used are probably the logistic regression and neural networks. Like all regression analyses, the logistic regression is used for forecasting. However, as opposed to simple and multiple linear regressions, the logistic regression has categorical data (yes or no, 0 or 1, etc.) as the output (see Graph 3).
Simply put, the logistic regression allows for determining whether an event is going to happen or not. Artificial neural networks, also referred to as connectionist systems, attempt to mimic the cognitive faculties of animal brains. Neural networks are capable of unsupervised learning, in which they are fed training data for pattern identification. In case the testing is successful, neural networks can proceed with conducting tasks without being explicitly programmed to do so (see Graph 4 for architecture of neural networks).
A prime example of the use of predictive modeling in finance is gauging the profitability of an investment. The success of an investment depends on the ability of an investor to predict the future movement of the prices on the market. Stanković, Marković and Stojanović (2015) developed an AI-based investment optimization strategy for emerging markets. The researchers used an LS-SVM (least-squares support vector machine) model to predict the trend of stock indices’ value. As compared to traditional statistical methods, the model put forward by Stanković et al. (2015) proved to be better at handling non-linear relationships between variables, which is characteristic for financial markets.
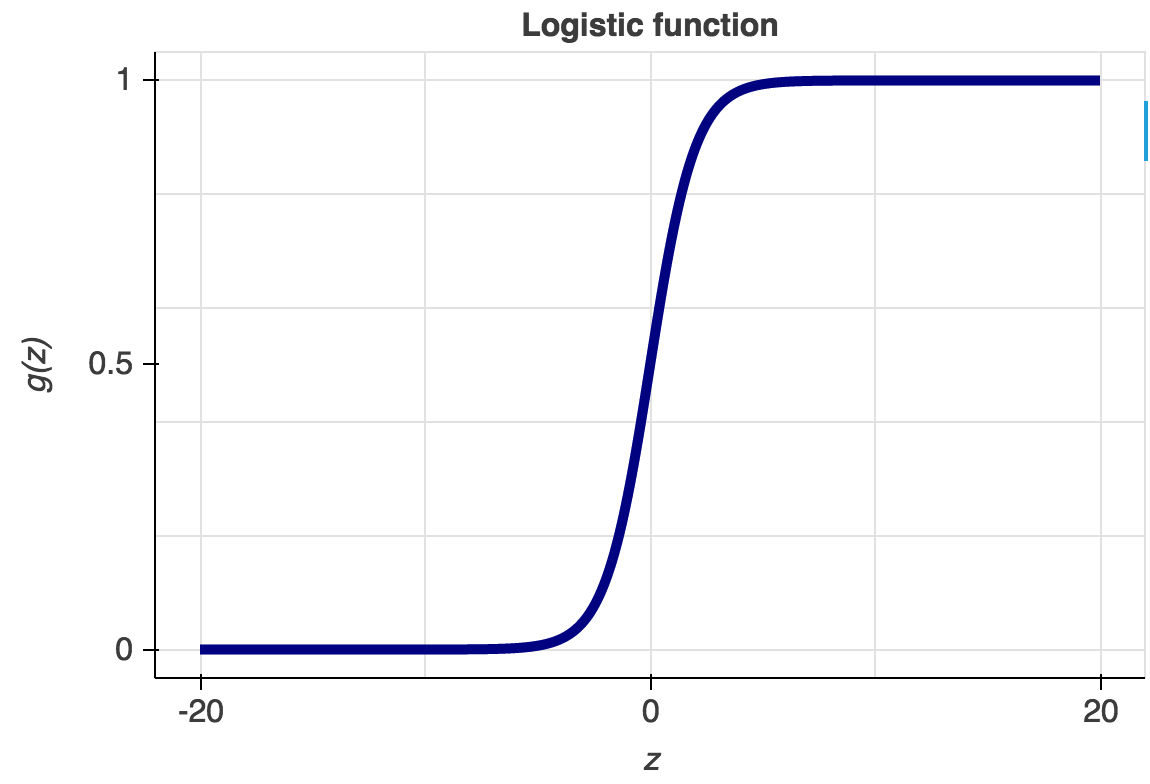
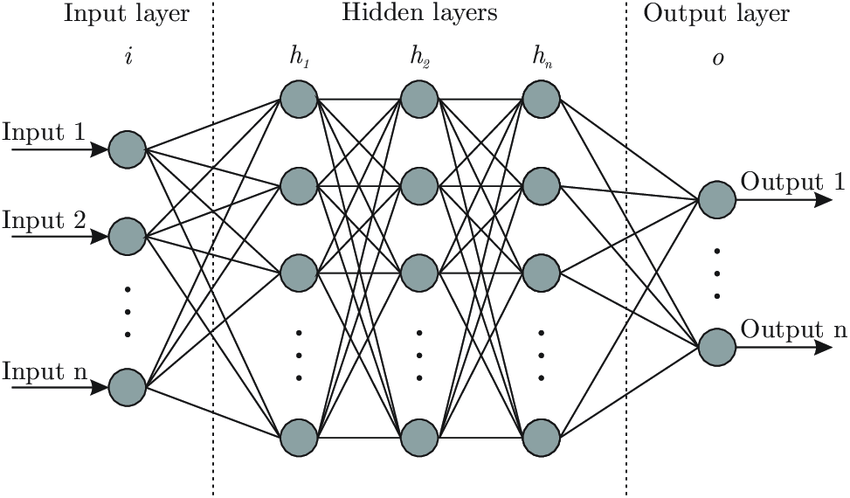
Medical diagnosis is one of the best examples of predictive analytics in healthcare, which has already made tangible progress. With millions of data entries accumulated over the past few years, the quantity of data is sufficient to develop extremely precise predictive models. Predictive modeling can be used in many cases, but the subfield that has arguably been gaining the most traction is predictive diagnosis. A prime example would be Q-Poc, a diagnostic tool developed by QuantumMDx, a UK medical device company, that uses predictive modeling to diagnose disease in under 20 minutes (Kourou, Exarchos, Exarchos, Karamouzis & Fotiadis, 2015). It has yet to find wider application in the UK and worldwide, but the prospects of its use are quite promising since Q-Poc has the potential to rectify improper diagnosis and reduce wait times.
Another excellent example of predictive modeling in medicine is diagnosing rare diseases that often go unnoticed in patients, leading to aggravation and multiple complications in the long run. In 2016, IBM announced collaboration with the Undiagnosed and Rare Diseases Center at the University Hospital in Marburg, Germany. The Center serves patient populations that have been to many specialists (some as many as 40) but who failed to receive a proper diagnosis. Artificial intelligence that employs wide databases and has a high degree of precision when detecting anomaly has the potential to help such patients.
Predictive modeling is on its way to be an indispensable part of cardiology as more methods find its application in the field. Awan, Bennamoun, Sohel, Sanfilippo and Dwivedi (2019) worked with Western Australian patients above 65 years that were hospitalised with heart failure between 2003 and 2008. The objective of the research was to predict hospital readmission or death within 30 days. To reach that objective, Awan et al. (2019) developed a multi-layer perceptron (MLP)-based approach and compared the results to those of other ML- and statistical methods. Out of 10,757 patients with heart failure observed by Awan et al. (2019), 23.6% were readmitted or passed away. When contrasting the new approach to other existing methods, the researchers concluded that their approach was superior in its sensitivity and specificity.
Another model for predicting mortality in patients with heart failure was put forward by Bowen, Diop, Jiang, Wu and Rudolph (2018). The independent variables, i.e. risk factors, pinpointed by the researchers included old age, ejection fraction, mean arterial pressure, pulse, brain natriuretic peptide, and others. Other risk factors used for building the model were hospitalization with length of stay over seven days in the past year and prior admission for palliative care.
Based on those variables, the model assigned each participant a score and classified him or her into one of the designated groups. The groups ranged from low-risk to very high and mortality risk groups. Bowen et al. (2018) concluded that while the mechanism was relatively simple, it showed a high degree of precision, which would allow for its integration into clinical practice. Data on the risk factors should be extracted from their medical records. Individuals that were assigned to high-risk groups are subject to discussion and counseling.
Hannun et al. (2019) applied the second predictive modeling method described in this section – neural networks – to arrhythmia detection in cardiology. The researchers saw an opportunity to refine diagnostic precision in using computerised electrocardiogram interpretation (ECG). Hannun et al. (2019) highlighted the fact that ECG data is widely available, and its volume allows for building predictive models that can become part of clinical practice. The researchers sought to fill in the knowledge gap that they had identified, namely, the lack of information regarding a wide variety of diagnostic classes in ECG analysis.
Hannun et al. (2019) developed a deep neural network (DNN) to outline 12 rhythm classes using over 90,000 single-lead ECGs from the monitoring devices of 53,000 patients. The results of the DNN were verified and approved by a consensus committee consisting of board-certified practicing cardiologists. By comparing and contrasting DNN and manual analysis, Hannun et al. (2019) concluded that the accuracy of their method surpasses that of the average cardiologist.
As any other models, predictive models should be subject to scrutiny and careful validation, especially given that health concerns should be treated with utmost seriousity. Typically, a predictive model must undergo three types of validation before it is ready for implementation:
- Model specification – selection of variables. In the case of cardiology, building a predictive model for a particular heart disease would require extensive background research. The objective of research would be to pinpoint risk factors that would be relevant for broader populations. Given that, different demographics have varying degrees of likelihood of suffering from heart disease, the model would need careful readjustment. Besides, modern science keeps making discoveries in the field of cardiology. Hence, it is only natural that any day, new evidence may appear that would need to be integrated into the predictive model;
- Model quantification: estimation of coefficients using the least squares method to build a regression equation (Ben-Moshe, 2016);
- Predictive ability: model performance. Yu et al. (2018) conducted a study with an intent to develop and validate a risk prediction model for peritoneal dialysis (PD) patients. As Yu et al. (2018) state, PD are at risk for developing cardiovascular disease, which has become the leading cause of death in the said patient population.The researchers applied three strategies for gauging model performance with objectivity:
- The sample was broken down into two groups (ratio = 60:40), with one group being tested for model derivation, and the other for model validation. In this way, the model was verified outside the initial group;
- Yu et al. (2018) used AUC-ROC (area under the ROC curve), which is independent of the change in the ratio of respondents;
- Lift and Gain charts: Yu et al. (2018) measured the model’s performance by computing the ratio between the results obtained with its use and without it.
Anomaly Detection
One of the top concerns of the financial sector is security threats that are increasing alongside the growing number of transaction users and third-parties. Fintech giants such as Adyen, Payoneer, Paypal, Stripe and Skrill are now heavily investing in security machine learning. The new tendency comes as no surprise as machine learning algorithms are considered fairly efficient at detecting frauds. A prime example would be banks implementing a technology that tracks and monitors hundreds upon hundreds parameters for every user account in real time. Based on the vast amounts of data, the system defines the typical patterns of each user’s behaviour.
Then, when someone is trying to make a transaction from an account that is being analyzed, the algorithm compares the attempted activity to that characteristic of that particular user. It has been shown that machine learning algorithms detect fraudulent activities with a high degree of precision.
The reason why machine learning is needed for ensuring the security of financial data lies in its proactive nature. It takes an algorithm a few seconds to detect an anomaly, which may help to prevent a crime from happening altogether. Post-factum detection would not be as helpful since it would take much more time to investigate the case. On the contrary, once an ML mechanism has identified suspicious account behaviour, it requires the user to take additional authentication steps. In some cases, an algorithm can block the transaction altogether for the sake of safety.
From a technical standpoint, the most commonly used methods for anomaly detection in data science are the following:
Density-based anomaly detection
Density-based anomaly detection is based on the k-nearest neighbors (KNN) algorithm. The key assumption of KNN is that normal data points constitute a dense neighborhood on the scatter plot while anomalies stray further away from it (Kou, Peng & Wang, 2014). The closest set of data points is located using a score that is calculated by using Euclidean, Manhattan, Minkowski, or Hamming distance (see Image 3). Alternatively, the local-outlier factor (LOF) can be used for pinpointing distance metric otherwise known as reachability distance (Tang & He, 2017).
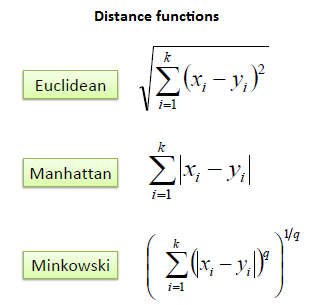
Clustering-based anomaly detection
Clustering is probably the most popular concept in the subfield of unsupervised machine learning. It is assumed that data points that showcase similitude will form groups or clusters when visualized on the scatter plot. The location and formation of clusters are determined by their distance from local centroids (Wiwie, Baumbach & Röttger, 2015). The most widely used clustering algorithm is based on K-means (see Image 4 for the formula). When put on a scatter plot, K-means forms “k” similar clusters of data points (see Graph 4 for visualization). Data instances that are estranged from these groups may be potentially marked as anomalies.
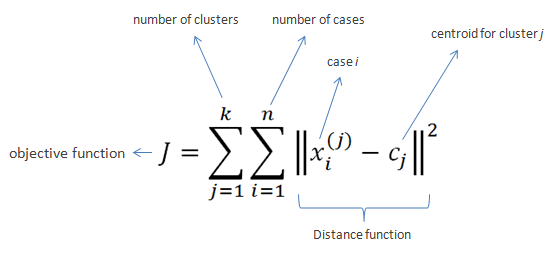
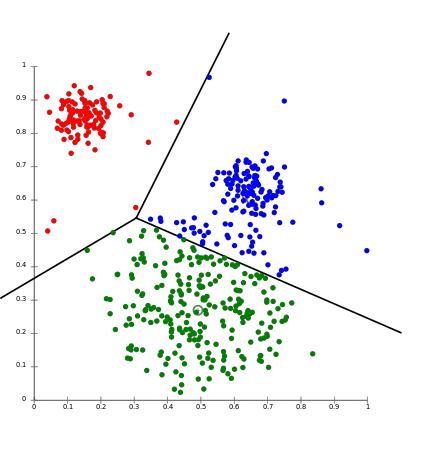
Support vector machine-based anomaly detection
Another commonly used technique for detecting anomalies is a support vector machine. A SVM is typically associated with supervised learning; however, some extensions allow for unsupervised problems in which training data is not labeled (Harris, 2015). The algorithm is communicated a soft boundary that is later used to form cluster of the normal data instances. By analyzing the testing instance, the algorithm becomes attuned to identifying abnormalities that stray from the learned region.
Detecting abnormalities can be used extensively in the healthcare section. However, as opposed to the financial sector, medicine would not be looking for fraudulent activities but for signs of disease. As mentioned earlier in this paper, cardiologists gather data from diagnostic imaging and monitoring. An example of K-means application in cardiological monitoring was recently presented in a paper by Zhu, Ding and Hao (2013). Zhu et al. (2013) put forward an innovative maximum margin clustering method with immune evolution (IEMMC). The objective of their study was to test the validity of the newly developed algorithm for diagnosing electrocardiogram (ECG) arrhythmias. The process entailed three essential steps: signal processing, feature extraction and the IEMMC algorithm for clustering of ECG arrhythmias.
The first step was processing raw ECG signal by an adaptive ECG filter based on wavelet transforms. The detection of the waveform of the ECG signal and feature extraction from ECG signal followed suit. The said features would later be clustered together with different types of arrhythmias by the IEEMC algorithm. Three criteria were used to gauge the efficiency of the IEMMC method: sensitivity, specificity and accuracy. As compared to other similar algorithms, the IEEMC showed better performance not only in clustering but also in global search and convergence faculties.
Another valuable piece of research employing clustering algorithms to detect cardiovascular abnormalities was presented by Sufi, Khalil and Mahmood (2011). In their study, Sufi et al. (2011) attempted to enhance the existing tele cardiological transmission system, Compressed Electrocardiology (ECG). The researchers pointed out a not-so-obvious pitfall of the widely used software: for detection mechanisms to process data, compressed ECG packets needed to be decompressed. The process would usually long enough to impact actual patient outcomes.
What stood out to Sufi et al. (2011) is that the algorithms did not need all the data encoded in the packages to function properly. Thus, the researchers employed an attribute selection method that targeted only the most essential properties encoded into a compressed ECG package. The next step entailed formation of normal and abnormal clusters using Expected Maximization (EM) clustering technique. The authors concluded that the new algorithm was reliable enough to be introduced in hospitals. Potentially, it would help emergency personnel to receive information on time and set on a rescue mission right away.
To validate an anomaly detection algorithm, it is recommended to split the dataset into training, cross-validation and testing set (60:20:20). A study by Chauhan and Vig (2015) describes the application of the said method to a real cardiological study. In their research, Chauhan and Vig (2015) focused on automating the process of analyzing electrocardiography (ECG) signals, which are used to estimate the health of the human heart. A time series signal, which is the result of cardiological examination, is often analyzed manually. Chauhan and Vig (2015) made an attempt at introducing a machine learning mechanism that would discern healthy signal from that characteristic of arrhythmia.
Chauhan et al. (2015) divided their dataset not into three but into four subsets: non-anomalous training set (sN ), non-anomalous validation set (vN ), combination of both anomalous and non-anomalous validation (vN+A) and test (tN+A) sets. A Recurrent Neural Network was trained to make forecasts using the non-anomalous training set (sN). At that, non-anomalous validation set was used for early stopping in case no anomaly was detected. The vN set was later analyzed for prediction error vectors that were fit to a Multivariate Gaussian using maximum likelihood estimation. After those steps have been undertaken, the Network was ready to work on vn+A set.
Discussion
For all its advantages, the use of artificial intelligence in finances and health care has its set of concerns. Some of them are technical issues, i.e. the current inability to translate innovative thought into action, while others pertain to ethical implications of big data mining and processing. In finance, the top concern is information security since financial information can be classified as sensitive. Ethical implications deal with data mining and collection techniques that raise a question whether those surveilled can actually consent to the said procedures (Xu, Jiang, Wang, Yuan and Ren, 2014).
As for healthcare, Panch, Mattie and Celie (2019) argue that it is still to early to call AI application a big success and that medical companies and healthcare providers should have their reservations. In particular, Panch et al. (2019) state that innovations such as AI and ML are not backed up by financial incentives as compared to traditional models. Moreover, AI and ML often have to be applied to fragmented data and incomplete databases, which undermines the validity of the results. This paper discussed some common algorithms in finances and the possibility of their application in the healthcare sector. Below are a critique of each of the discussed method and a preliminary consensus on its viability.
Credit scoring
As of now, the only sector where credit scoring is widely applied is finances. Yet, even in its main domain, credit scoring systems do not escape well-deserved criticism. In the absence of actual formulas that have never gone public, citizens cannot manage their score in any meaningful way. Thus, if an individual considers their score unfair or biased, there is no point of reference that they could use to appeal to the authority. For this reason, traditional, automated credit scoring tools have been considered problematic for an extended period of time.
As of now, the situation is likely to become even more complicated due to the recent advent of new big-data credit scoring products. The startup scene has seen an explosion of businesses that offer even more sophisticated solutions that treat all data as credit data (Hurley & Adebayo 2016). Information is often mined from consumers’ offline and online activities that provide companies with variables that go far beyond those known to the general public. In a way, it means a certain determinism of how a person’s life might unroll.
The top concerns of the credit scoring use in finance are likely to manifest themselves in the healthcare section as well and even more so. Ultimately, a health credit scoring system is likely to operate on a reward vs. punishment basis. Again, the criteria for being appraised or ostracised by the system will be predetermined by the industry’s giants, and consumers are unlikely to have a say in this conversation.
Moreover, a credit scoring system might have a detrimental effect on the most vulnerable demographics. If an individual already suffers from an addiction, and their living environment does not predispose them for making better choices, a low credit score will only further victimise them. In the long run, the situation will become a vicious circle spiraling out of control: the less access a struggling individual has to healthcare, the poorer their health choices and habits become (Mihai, Ţiţan, & Manea, 2015).
A vicious circle of poverty is not a sheer assumption. For instance, Carvalho, Meier and Wang (2016) and Bernheim, Ray and Yeltekin (2015).talk at length about the association between poverty and poor decision-making faculties. The researchers argue that subpar standards of living and acute financial problems make individuals so preoccupied with erratic thinking that they can no longer do long-term planning. Drawing on the research by Carvalho et al. (2016), it is safe to assume that an impoverished individual is more likely to pay regard to a problem at hand than to a score that considers longitudinal data.
Besides, if the variables used in the scoring algorithm ever make it to the public, individuals may as well start concealing health information in order to rectify their scores. This will mean that fewer people will be referred to the respective institutions to receive help, therefore, adding to the national burden of disease. In summation, while credit scoring systems seem to function in the financial sector, even though not without criticism, a similar system might be detrimental for health care.
Aside from ethical considerations, there is also a technical side to the subject matter that can also prove to be problematic. VantageScore experts discuss overlay criteria that may manifest themselves when various frameworks are applied to score users. In finance and namely, in credit, overlay means imposing rules on top of the bare minimum requirements for receiving a loan. In the context of medicine, this would mean healthcare facilities developing their own scoring criteria on top of those prescribed by the state. One may readily imagine that applying two or more frameworks simultaneously may complicate the situation. VantageScore (2012) put forward three key principles for scoring system validation that can be extrapolated to find a use in the healthcare sector as well.
To validate a scoring system, developers need to define the baseline default rates that would serve as a point of reference. This baseline will be used to gauge the model’s performance and can be readjusted to fit a user’s profile and treatment strategy. To calculate the baseline metrics for a scoring system, the overlay matrix should be applied to a data set for which scores are already known. If applied correctly, the output will be the expected default rates for later use in validating.
The next step would entail separation of overlay segments. Each overlay segment is to be treated as a unique portfolio. However, it should be noted that if segments showcase inconsistent performance across the board, there might be a problem with the overlay strategy itself. Lastly, VantageScore (2012) experts point out that the overlay strategy itself is subject to analysis. For this reason, it should be treated as an additional variable.
Predictive Modeling
As any other statistical method, predictive modeling has its limitations. Dismissing the shortcomings of predictive diagnosis in healthcare may jeopardise patients’ lives, therefore, the disadvantages need to be addressed. Bernard (2017) discusses at length the implications of predictive analysis in healthcare. While he acknowledges its potential to ramp up the efficiency of work processes, the researcher takes issue with two particular aspects of its use:
- Theoretical framework. To construct a viable model and assign scores to certain symptoms / phenomena, researchers need to use a valid theoretical framework. Prediction is based on pinpointing causation – a relationship that may be unique for each particular disease and that should be studied closely to manage risks. Bernard (2017) argues that not every prognostic study respects quality criteria, in particular the representativeness of the patients and the quality of the data. Fragmented databases and studies with compromised validity make predictive modeling challenging;
- Routine use. According to Altmann-Richer (2018), a predictive score should only be considered routinely if it has been validated in an independent sample. It implies that a medical facility cannot rely solely on its own database as the sampling may be fairly biased. Instead, a predictive model should on external sources of information and evidence-based practices;
Ratner (2017) points out one more issue with predictive modeling validation, which may especially pertain to the healthcare. Predictive models should undergo three degrees of validation: apparent (own sample), internal (own population), and external (external population). For this, however, there should exist well-developed, comprehensive databases apt not only for internal use but also for sharing information. As of now, health data is often fragmented, and its quality when obtained from different sources may vary greatly. Therefore, even if a predictive modeling algorithm is impeccable, there are not often many opportunities to validate it.
Anomaly Detection
Anomaly detection allows for anomaly identification in straightforward cases. However, when it comes to more complicated problems, some techniques may prove inefficient. If the data contains noise that is similar to abnormal behaviour, it is fairly challenging to validate conclusions (Ahmed, Mahmood and Hu, 2016). The boundary between normal and abnormal behaviour is often not precise: for instance, cardiology symptoms may vary greatly within the norm. One person’s cardiological patterns may be a cause for concern while similar patterns in another person may as well be non-threatening. These particularities are often only known when a health worker spends enough time with a patient and puts in thought in defining individual criteria of health. An algorithm, on the other hand handles explicit data and may fail to consider individual discrepancies when making diagnoses or giving recommendations.
Another issue one may take with anomaly detection in health care is changes in the definition of normal and abnormal (Ahmed et al., 2016). Since as any other medical subfield, cardiology becomes more evidence-based, i.e. translates scientific findings into daily practice, the norm may as well be subject to change. For anomaly detection mechanisms, this trend may mean non-applicability of the threshold on moving average. Lastly, some patterns are based on seasonality, which requires more sophisticated methods to decompose data into multiple trends.
Conclusion
Artificial intelligence and machine learning have promising prospects of application in multiple fields, including finance and health care. The analysis has shown, each of the commonly used methods in finances is applicable to health care. Some of the techniques are already used while the use of which is only theorised and has yet to be tested for efficiency. The most viable AI and ML applications for health care that can be borrowed from finances are predictive modeling and anomaly detection. However, their validation may prove challenging: both predictive modeling and anomaly detection may be compromised by the incompleteness of existing health care databases.
Besides, as modern medicine makes progress, the models might need readjustment to integrate new findings concerning thresholds between anomaly and normality and risk factors. Lastly, the least applicable model is probably a credit scoring system. While in finance, one’s credit score determines their social standing and financial prospects, in health care, failing to rectify a score may mean decreased survivability. From a technical standpoint, a health scoring model may run into the issue of overlaying criteria.
Reference List
Ahmed, M, Mahmood, AN, and Hu, J 2016, ‘A survey of network anomaly detection techniques’, Journal of Network and Computer Applications, vol. 60, pp.19-31.
Altmann-Richer, L 2018, Using predictive analytics to improve health care demand forecasting. Web.
Andjelic, L 2019, These fintech statistics show an industry on the rise. Web.
Awan, SE, Bennamoun, M, Sohel, F, Sanfilippo, FM & Dwivedi, G 2019, ‘Machine learning‐based prediction of heart failure readmission or death: implications of choosing the right model and the right metrics’, ESC Heart Failure, vol. 6, no. 2, pp.428-435.
Bahnsen, AC, Aouada, D & Ottersten, B 2014, ‘Example-dependent cost-sensitive logistic regression for credit scoring,’ In 2014 13th International Conference on Machine Learning and Applications, IEEE, pp. 263-269.
Ben-Moshe, D 2016, Identification and estimation of coefficients in dependent factor models. Web.
Bernard, A 2017, ‘Clinical prediction models: a fashion or a necessity in medicine?’ Journal of thoracic disease, vol. 9, no. 10, pp. 3456–3457.
Bernheim, BD, Ray, D & Yeltekin, Ş 2015, ‘Poverty and self‐control’, Econometrica, vol. 83, no. 5, pp.1877-1911.
Bleustein, C, Rothschild, DB, Valen, A, Valatis, E, Schweitzer, L & Jones, R 2014, ‘Wait times, patient satisfaction scores, and the perception of care’, The American Journal of Managed Care, vol. 20, no. 5, pp.393-400.
Bowen, GS, Diop, MS, Jiang, L, Wu, WC & Rudolph, JL 2018, ‘A multivariable prediction model for mortality in individuals admitted for heart failure’, Journal of the American Geriatrics Society, vol. 66, no. 5, pp.902-908.
Buch, VH, Ahmed, I & Maruthappu, M 2018, ‘Artificial intelligence in medicine: current trends and future possibilities’, The British Journal of General Practice : the journal of the Royal College of General Practitioners, vol. 68, no. 668, pp. 143–144.
Cambria, E & White, B 2014, ‘Jumping NLP curves: a review of natural language processing research’, IEEE Computational Intelligence Magazine, vol. 9, no. 2, pp.48-57.
Carvalho, LS, Meier, S & Wang, SW 2016, ‘Poverty and economic decision-making: evidence from changes in financial resources at payday’, American Economic Review, vol. 106, no. 2, pp.260-84.
Centers for Disease Control and Prevention 2017, Heart disease facts. Web.
Centers for Disease Control and Prevention 2015, Heart disease risk factors. Web.
Chauhan, S & Vig, L 2015, ‘Anomaly detection in ECG time signals via deep long short-term memory networks’, In 2015 IEEE International Conference on Data Science and Advanced Analytics (DSAA), pp. 1-7.
Columbus, L 2019, Why AI Is The future of financial services. Web.
Dinov, ID 2016, ‘Methodological challenges and analytic opportunities for modeling and interpreting Big Healthcare Data’, Gigascience, vol. 5, no. 1, p. 12.
Fabian, B, Ermakova, T & Junghanns, P 2015, ‘Collaborative and secure sharing of healthcare data in multi-clouds’, Information Systems, vol. 48, pp. 132-150.
Fatima, M, Anjum, AR, Basharat, I & Khan, SA 2014, ‘Biomedical (cardiac) data mining: extraction of significant patterns for predicting heart condition’ In 2014 IEEE Conference on Computational Intelligence in Bioinformatics and Computational Biology, pp. 1-7.
Hannun, AY, Rajpurkar, P, Haghpanahi, M, Tison, GH, Bourn, C, Turakhia, MP & Ng, AY 2019, ‘Cardiologist-level arrhythmia detection and classification in ambulatory electrocardiograms using a deep neural network’, Nature Medicine, vol. 25, no. 1, p.65.
Harris, T 2015, ‘Credit scoring using the clustered support vector machine’, Expert Systems with Applications, vol. 42, no. 2, pp. 741-750.
Hurley, M & Adebayo, J 2016, ‘Credit scoring in the era of big data’, Yale Journal & Technology, vol. 18, p.148.
Hirschberg, J & Manning, CD 2015, ‘Advances in natural language processing’, Science, vol. 349, no. 6245, pp.261-266.
Jiang, F et al. 2017, ‘Artificial intelligence in healthcare: past, present and future’, Stroke and Vascular Neurology, vol. 2, no. 4, pp.230-243.
Johnson, KW et al. 2018, ‘Artificial intelligence in cardiology’, Journal of the American College of Cardiology, vol. 71, no. 23, pp.2668-2679.
Kadi, I, Idri, A & Fernandez-Aleman, JL 2017, ‘Knowledge discovery in cardiology: a systematic literature review’, International Journal of Medical Informatics, vol. 97, pp.12-32.
Kayyali, B, Kuiken, SV, & Knott, D 2013, The big-data revolution in US health care: accelerating value and innovation. Web.
Kou, G, Peng, Y & Wang, G 2014, ‘Evaluation of clustering algorithms for financial risk analysis using MCDM methods’, Information Sciences, vol. 275, pp.1-12.
Kourou, K, Exarchos, TP, Exarchos, KP, Karamouzis, MV & Fotiadis, DI 2015, ‘Machine learning applications in cancer prognosis and prediction’, Computational and Structural Biotechnology Journal, vol. 13, pp.8-17.
Kwong, RY, Jerosch-Herold, M & Heydari, B (eds.) 2018, Cardiovascular magnetic resonance imaging, Springer, New York.
Mahajan, SM, Heidenreich, P, Abbott, B, Newton, A & Ward, D 2018, ‘Predictive models for identifying risk of readmission after index hospitalization for heart failure: a systematic review’, European Journal of Cardiovascular Nursing, vol. 17, no. 8, pp.675-689.
Mihai, M, Ţiţan, E & Manea, D 2015, ‘Education and poverty’, Procedia Economics and Finance, vol. 32, pp. 855-860.
Mozaffarian D et al. 2015, ‘Heart disease and stroke statistics—2015 update: a report from the American Heart Association’, Circulation, vol. 131, pp. 29-32.
Panch, T, Mattie, H & Celi, LA 2019, ‘The “inconvenient truth” about AI in healthcare’, NPJ Digital Medicine, vol. 2.
Raghupathi, W & Raghupathi, V 2014, ‘Big data analytics in healthcare: promise and potential’, Health Information Science and Systems, vol. 2, no. 1, p.3.
Ratner, B 2017, Statistical and machine-learning data mining : techniques for better predictive modeling and analysis of big data, Chapman and Hall/CRC, London..
Sisko, AM et al. 2019, ‘National health expenditure projections, 2018–27: economic and demographic trends drive spending and enrollment growth’, Health Affairs, vol. 38, no. 3, pp.491-501.
Sufil, F, Khalil, I & Mahmood AN 2011, ‘A clustering based system for instant detection of cardiac abnormalities from compressed ECG’, Expert Systems with Applications, vol. 38, no. 5, pp. 4705-4713
Stanković, J, Marković, I & Stojanović, M 2015, ‘Investment strategy optimization using technical analysis and predictive modeling in emerging markets’, Procedia Economics and Finance, vol. 19, pp. 51-62.
Stubbs, A, Kotfila, C, Xu, H & Uzuner, Ö 2015, ‘Identifying risk factors for heart disease over time: Overview of 2014 i2b2/UTHealth shared task Track 2’, Journal of Biomedical Informatics, vol. 58, pp.S67-S77.
Tang, B & He, H 2017, ‘A local density-based approach for outlier detection’, Neurocomputing, vol. 241, pp.171-180.
Tao, S, Hoffmeister, M & Brenner, H 2014, ‘Development and validation of a scoring system to identify individuals at high risk for advanced colorectal neoplasms who should undergo colonoscopy screening’, Clinical Gastroenterology and Hepatology, vol. 12, no. 3, pp.478-485.
Tirinato, JA et al. 2015, Medical data acquisition and patient management system and method, U.S. Patent 9,015,055.
VantageScore 2012, Validating a credit score model in conjunction with additional underwriting criteria. Web.
Velupillai, S, Mowery, D, South, BR, Kvist, M & Dalianis, H 2015, ‘Recent advances in clinical natural language processing in support of semantic analysis’, Yearbook of Medical Informatics, vol. 24, no. 1, pp.183-193.
Walker, T 2018, How predictive modeling, big data analytics curb health costs in China. Web.
Wiwie, C, Baumbach, J & Röttger, R 2015, ‘Comparing the performance of biomedical clustering methods’, Nature Methods, vol. 12, no. 11, p.1033.
Xu, L, Jiang, C, Wang, J, Yuan, J & Ren, Y 2014, ‘Information security in big data: privacy and data mining’, Ieee Access, vol. 2, pp. 1149-1176.
Yu, D, Cai, Y, Chen, Y, Chen, T, Qin, R, Zhao, Z & Simmons, D 2018, ‘Development and validation of risk prediction models for cardiovascular mortality in Chinese people initialising peritoneal dialysis: a cohort study’, Scientific Reports, vol. 8, no. 1, p.1966.
Zhu, B, Ding, Y, & Hao, K 2013, ‘A novel automatic detection system for ECG arrhythmias using maximum margin clustering with immune evolutionary algorithm’, Computational and Mathematical Methods in Medicine, vol. 2013, pp. 1-8.